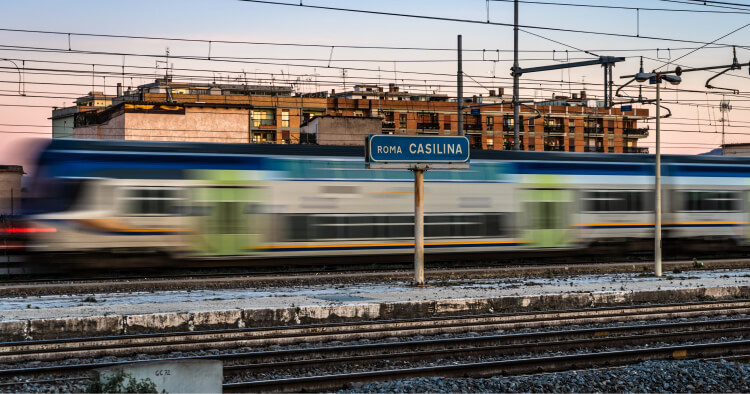
Implementing a Data Lifecycle Management Strategy
Nov 11, 2024
Data remains the driving force behind innovation and business growth. However, managing this wealth of information effectively requires a forward-thinking strategy. Without a structured approach, companies may struggle with inconsistent, redundant, or inaccurate data, leading to operational inefficiencies and poor decision-making.
What is Data Management?
At its foundation, data management involves the policies and procedures that oversee an organization’s data throughout its lifecycle. This includes data collection, storage, processing, and analysis. When managed properly, data becomes a reliable asset that supports accurate decision-making and enhances operational efficiency. Without proper management, however, data can become disorganized and lead to suboptimal business outcomes.
A Data Lifecycle Management (DLM) strategy outlines the process for handling data from the moment it is created to when it is no longer needed and can be safely deleted. This strategy not only helps maintain the integrity and security of data but also ensures compliance with relevant regulations.
A robust DLM strategy emphasizes data protection and disaster recovery, particularly as the rapid growth of data attracts more malicious actors. By having an effective data recovery plan in place, businesses can mitigate the severe impacts on their bottom line and reputation in the event of a disaster.
A critical component of any DLM strategy is creating a tailored retention schedule. This document should outline how long different types of data—whether physical or digital—should be stored. It must also take into account various data types, such as personally identifiable information (PII), intellectual property (IP), and financial records. The retention period may vary depending on the nature of the data and specific regulations like GDPR.
The Scale of Data
Data volumes can range significantly depending on the size and industry of an organization. For example, a mid-sized company might manage between 10 and 50 terabytes of data, including customer records and operational data. Larger organizations, particularly in sectors like finance or technology, often deal with petabytes of data, highlighting the importance of efficient storage and management practices.
To visualize, 50 terabytes is roughly equivalent to 12.5 million four-minute songs or around 10 million high-resolution photos. If stored on DVDs, this amount of data would require about 10,000 discs, demonstrating the immense storage needs that modern companies must manage.
Data Versus Information
Data and information are related but distinct concepts. Data refers to raw facts and figures without context, such as numbers, dates, and strings of text. For example, a list of temperatures recorded over a week is data.
Information, on the other hand, is data that has been processed, organized, or structured to provide meaning. For instance, analyzing that temperature data to determine the average heat for the week transforms data into information that can inform decisions. In essence, data becomes information when it is interpreted and contextualized.
Information Lifecycle Management (ILM) is often used interchangeably with Data Lifecycle Management (DLM), but while both are integral to data management practices, they are distinct.
DLM focuses on managing file-level data, organizing files based on type, size, and age. In contrast, ILM manages the individual pieces of data within those files, ensuring accuracy and timely updates. This includes user information such as email addresses and account balances.
Pillars of Effective Data Management
Governance: This provides the framework for managing data by establishing policies, standards, and accountability. It defines access permissions and ensures regulatory compliance.
Quality: Maintaining high-quality data—meaning data that is accurate, complete, and reliable—is vital. Regular audits, data cleansing, and validation help maintain data quality.
Integration: As organizations expand, data is often spread across multiple systems. Data integration involves consolidating this information into a unified system to enable comprehensive analysis.
Security: With the growing threat of cyberattacks, protecting data from unauthorized access is paramount. Encryption, access control, and routine security audits are key measures for safeguarding sensitive information.
Analytics:Transforming raw data into actionable insights is the ultimate goal of data management. Tools like artificial intelligence and machine learning can enhance an organization’s analytical capabilities, driving innovation and competitive advantage.
Balancing Data Retention with Security, Performance
Many organizations adopt a “keep it just in case” approach to data, holding onto historical data that may never be useful. This can result in significant costs and risks, as excessive data retention and storage open the doors to privacy and security issues. Organizations must ensure the security and privacy of growing volumes of confidential information, as mandated by industry regulations and government requirements, even in development and test environments.
Moreover, large data volumes can lead to slow response times and degraded performance, becoming major application issues. Continued data growth, if left unchecked, will stretch resources beyond capacity, negatively impacting critical reporting and query response times. Implementing intelligent management of dormant historical data is essential to avoid these potentially business-halting issues and to control operational costs, which often consume most of the data management or warehousing budget.
Experience Operational Efficiencies
An effective data management strategy helps organizations streamline their operations, enhance security, and maintain compliance with regulatory standards. By developing strong data governance, ensuring data quality, and using modern tools for analysis, companies can transform their data from a challenge into a strategic asset. Managing the lifecycle of data from creation to deletion not only reduces risk but also optimizes productivity and decision-making.
Overall, DLM is essential for protecting data, reducing costs, ensuring compliance, and enhancing the quality and efficiency of business operations.